“To me, it seems that those sciences are vain and full of errors which are not born of experience, mother of all certainty, first-hand experience which in its origins, or means, or end has passed through one of the five senses. And if we doubt the certainty of everything which passes through the senses, how much more ought we to doubt things contrary to these senses – ribelli ad essi sensi – such as the existence of God or of the soul or similar things over which there is always dispute and contention. And in fact, it happens that whenever reason wants men to cry out against one another, which does not happen with certainties. For this reason, we shall say that where the cry of controversy is heard, there is no true science, because the truth has one single end and when this is published, the argument is destroyed forever”
— Leonardo da Vinci, Trattato della pittura
Population Dynamics
Population dynamics models discussed in Weibull (1994) are reviewed next. First, various cases of population dynamics consistent with the conclusions from the previous section are summarized. Then, the most appropriate population dynamics model is utilized to demonstrate collective behavioral intelligence in the subsequent blog on the Singularity.
Key conclusions from the preceding chapter include:
- The mixed strategies representing average behavior in each population constitute an equilibrium point, albeit an imperfect one given imperfect information utilization and stability of strategy frequencies (Weibull, J.W. 1994).
- The aggregate process of strategy adaptation across individuals can be approximated as deterministic flows described by differential equations, if strategy choices are updated randomly yet frequently (Weibull, J.W. 1994).
Weibull, J.W. (1994) suggests Nash equilibria could represent stationary or dynamically stable states in dynamic models of bounded rationality-based strategy adaptation in large interacting populations. He discusses mathematical population dynamics models like replicator dynamics that emerge from “social evolution”-based processes. Weibull attempts to reinterpret Nash’s mass action interpretation using various population dynamics frameworks.
“Nash Equilibria could be identified as stationary, or perhaps dynamically stable, population states in dynamic models of boundedly rational strategy adaptation in large strategically interacting populations” (Weibull, J.W. 1994)
Nash’s mass action interpretation, as described previously, involves accumulation of empirical information on relative payoffs of different strategies. Nash’s M.A.I as explained in the preceding section describes “memory and expectation formation – the participants are supposed to accumulate empirical information on the relative advantages of the various pure strategies at their disposal.” Thus, population dynamics with memory and “innovation” may best capture Nash’s mass action interpretation. The population dynamics model most capable of representing such behavior is therefore selected to model strategic interactions with embedded equilibrium states.
Cases of Population Dynamics
Standard Description

In the context of population dynamics, the standard description assumes that individuals do not employ novel strategies but instead switch between existing strategies with their corresponding payoffs or performances. The growth rate function represents the growth rate of “α-strategists” within a population state ‘s’ and player populace ‘i’. It is important to note that all solutions in S remain in S, meaning that there is no deviation from the pure strategy profile adopted by all players. This scenario is similar to a distribution of available strategies that does not constitute a Nash equilibrium because players who individually adopt a pure strategy will continue to do so indefinitely, as explained by Weibull, J.W (1994).
In essence, the standard description of population dynamics assumes that individuals do not adapt or innovate, but instead stick to their pre-existing strategies, and the growth rate function reflects the growth rate of a specific group of strategists within a population state. Additionally, all solutions in S remain in S, implying that there is no deviation from the pure strategy profile adopted by all players, which is similar to a Nash equilibrium.
Imitative Adaptation
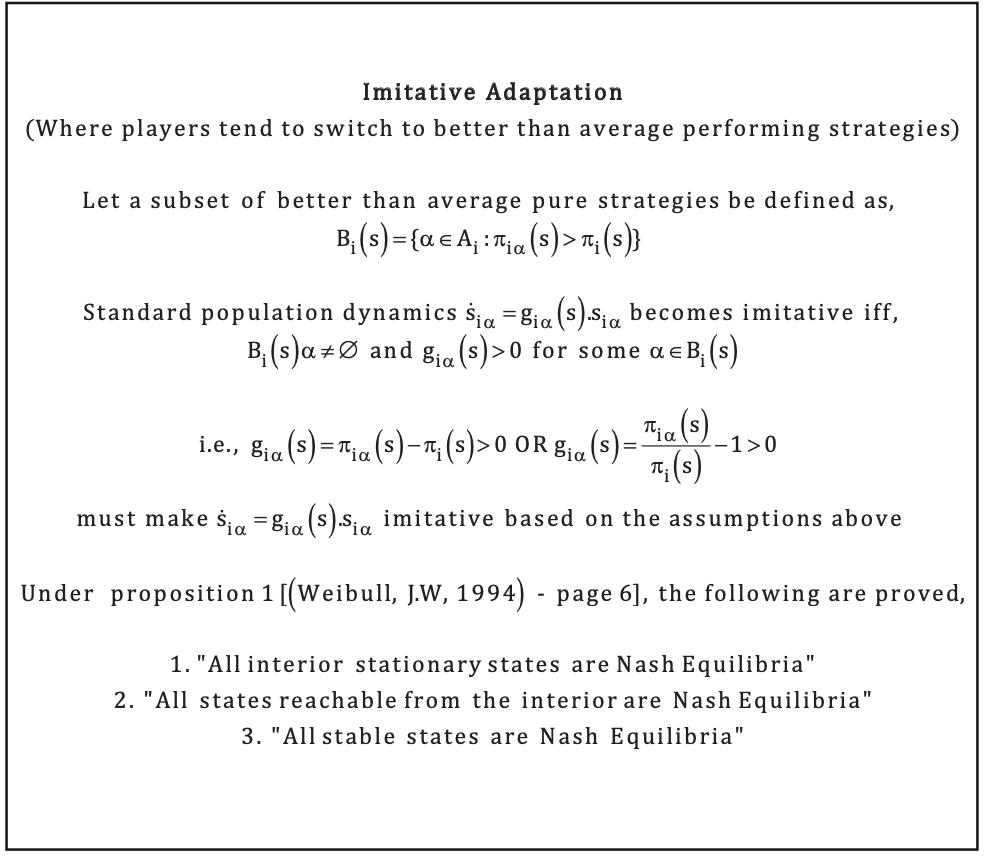
In the context of imitative adaptation, innovative adaptation assumes that if a pure strategy yields an above-average payoff within a player population, its growth rate will be positive. Consequently, strategies that perform better than average will increase in population share. Individuals switch to strategies that perform better, and although requirements to model swarm intelligence are found, they are limited in scope. The properties of this scenario, as defined by Weibull (1994), include stationarity, stability, and reachability. Stationarity states that all solutions in S remain in S, stability indicates that small perturbations do not lead the population state away, and reachability or reachable from the interior means that a state is the limit to some interior solution. Notably, all interior stationary states, stable states, and reachable states are Nash equilibria, as proven by Weibull (1994).
In summary, the description of imitative adaptation assumes that individuals switch to better-performing strategies, leading to growth in population share for above-average strategies. The properties of this scenario include stationarity, stability, and reachability, and all interior stationary states, stable states, and reachable states are Nash equilibria.
Innovative Adaptation (Experimentation or Mutation)
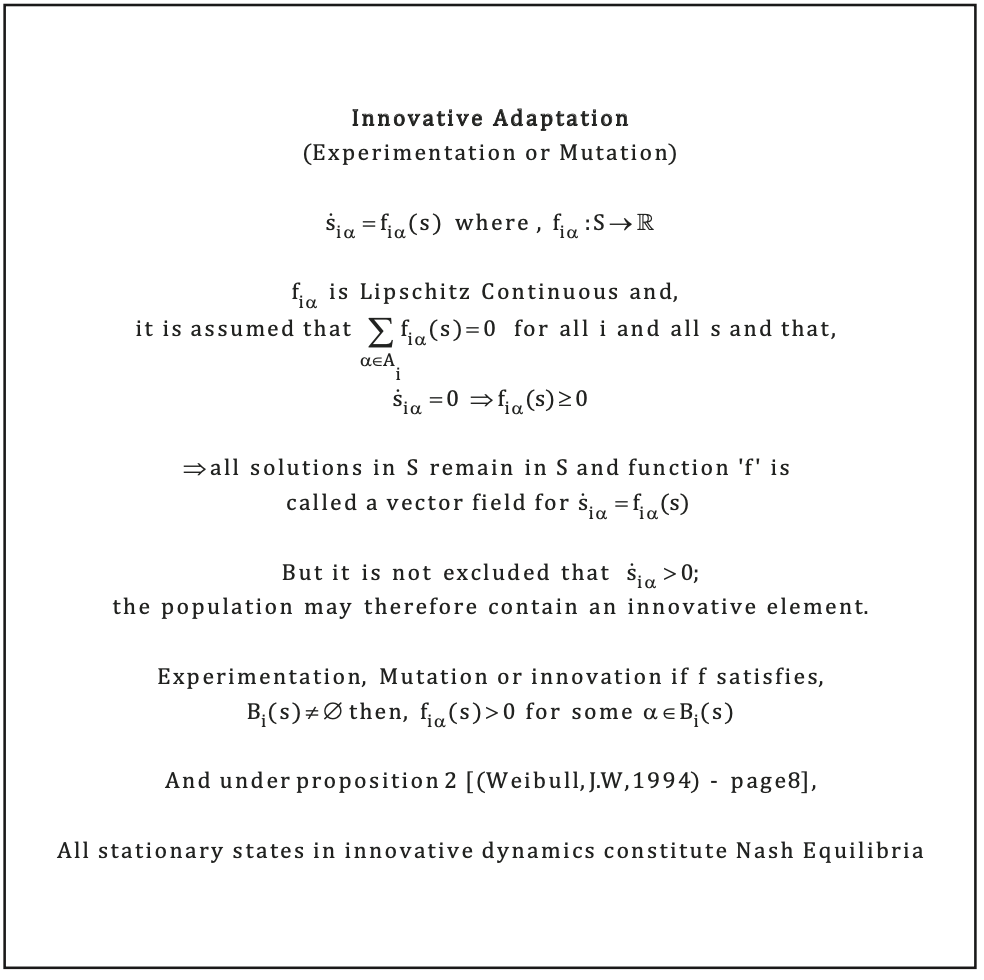
Innovative adaptation incorporates several critical capabilities for modeling strategic interactions, including the ability for extinct pure strategies to randomly reenter the population through mutation or experimentation (Weibull, 1994). This enables persistent diversity and inventiveness. Additionally, new and modified strategies can emerge through such experimentation, facilitating adaptive evolution. As proven by Weibull (1994), all stationary states constitute Nash equilibria, upholding important theoretical properties. Further enabling complex modeling, the expanded strategy space matches requirements for representing swarming behavior over an extensive, determinable state space.
In total, innovative adaptation provides critical modeling capabilities including resuscitating extinct strategies, generating inventive new strategies, ensuring Nash equilibrium properties, and allowing complex swarm modeling over an enlarged state space. These features significantly enhance the representation of adaptive multi-agent strategic interactions.
Innovative Adaptation with Memory (Population-Payoff-Perception)
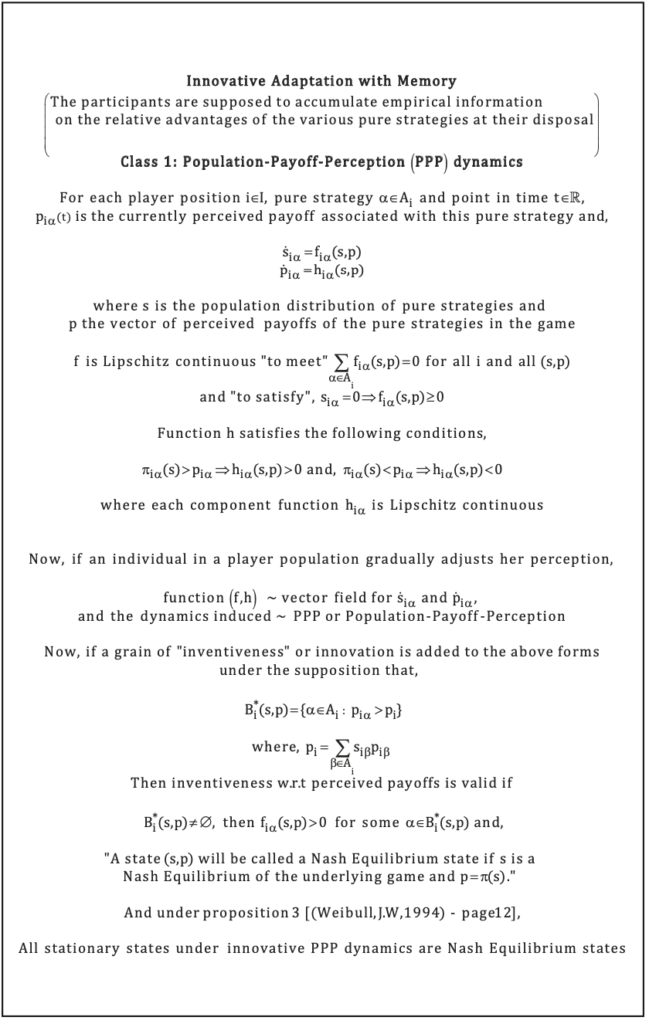
The innovative adaptation with memory (PPP) incorporates relative payoff perception, whereby strategies’ currently perceived payoffs are adjusted upward or downward depending on whether their realized payoffs exceed or fall short of the perception (Weibull, 1994). This enables continuous tuning of strategy usage. Specifically, pure strategies with above-average perceived payoffs increase their population share until superseded by even better-performing pure strategies with higher perceived payoffs (Weibull, 1994). As Weibull (1994) proves in Proposition 3, all PPP stationary states constitute Nash equilibria, preserving important theoretical properties. Additionally, the responsive payoff-based strategy tuning matches requirements for modeling swarm intelligence.
In summary, the key capabilities provided by innovative adaptation with memory include relative payoff perception, continuous payoff-driven strategy tuning, theoretical guarantees on stationary states, and suitability for swarm modeling. Through these mechanisms, innovative adaptation with memory facilitates effective modeling of adaptive strategic interactions between agents.
Innovative Adaptation with Memory (Perceived-Population-Shares)
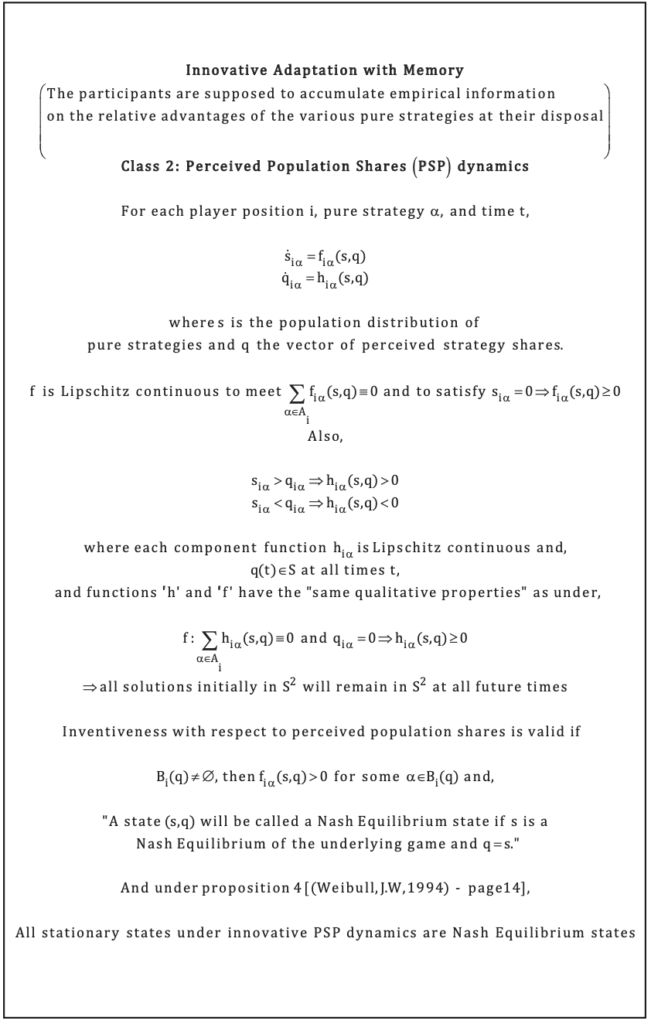
The innovative adaptation with memory incorporating payoff and share perception (PSP) enables players to leverage knowledge of the game’s payoff function and perceived population shares of strategies (Weibull, 1994). With this information, pure strategies yielding above-average payoffs given current perceived population shares will increase their share in the actual population. As Weibull (1994) proves in Proposition 4, all PSP stationary states constitute Nash equilibria, upholding important theoretical guarantees. Additionally, the responsive, payoff-driven strategy adaptation facilitated by payoff and share perception matches requirements for effectively modeling swarm intelligence.
In summary, innovative adaptation with memory via PSP provides players with payoff function knowledge and perceived population shares to guide beneficial pure strategy expansion. The key features include theoretically-assured Nash equilibrium stationary states and suitability for swarm modeling. Through payoff-based adaptation driven by share perception, innovative adaptation with memory enables sophisticated modeling of strategic multi-agent interactions.
Summary
1. Standard population dynamics:
- Individuals do not innovate, only switch between existing strategies
- Growth rate function tracks growth of strategist groups
- Solutions remain within initial strategy space S
- Similar to non-Nash equilibrium distribution of strategies
2. Imitative adaptation:
- Individuals switch to better-performing strategies
- Above-average strategies increase in population share
- Exhibits stationarity, stability, and reachability properties
- Interior stationary/stable/reachable states are Nash equilibria
3. Innovative adaptation:
- Extinct strategies can reenter through mutation
- New strategies can emerge through experimentation
- Stationary states are Nash equilibria
- Expanded strategy space enables complex swarm modeling
4. Innovative adaptation with memory (PPP):
- Relative payoff perception continuously tunes strategies
- Above-average payoff strategies expand
- Stationary states are Nash equilibria
- Matches swarm modeling requirements
5. Innovative adaptation with memory and share perception (PSP):
- Leverages payoff function knowledge and perceived shares
- Above-average payoff strategies expand given shares
- Stationary states are Nash equilibria
- Payoff-based adaptation enables swarm modeling
In summary, the standard description is limited as individuals cannot innovate, while the subsequent scenarios incorporate increasing adaptation capabilities. PSP provides the most sophisticated modeling via payoff knowledge and share perception guiding strategy innovation and population dynamics.